We recently worked with Parkinson’s UK to improve their data quality and help inform the data architecture. The first thing we did was to conduct a landscape analysis – a rigorous process of data analysis and documentation.
The landscape analysis played a vital role in helping the charity look at the current state of data quality. As well as looking at the data itself, the landscape analysis looked at data processes, integrations, and platforms used. We used a selection of tools and techniques to analyse the data structure, content and relationships.
Here are the 5 key stages of the landscape analysis, each which helped Parkinson’s UK improve their data quality:
1. Better understanding of their data infrastructure
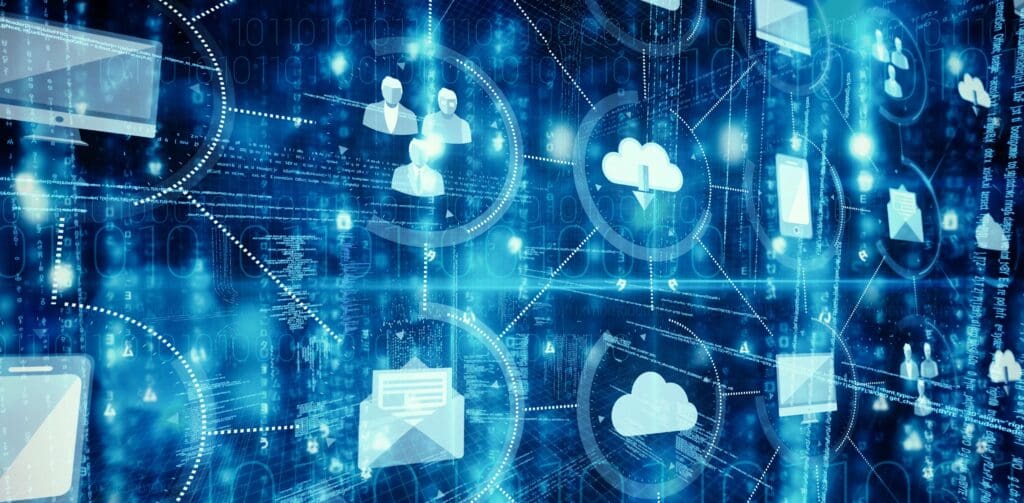
Our first step in the landscape analysis was to deploy a Headline Data Catalogue. A Headline Data Catalogue is a high-level catalogue of the readily available data looking at content, format and usability. This provided us with a clear picture of what data has been collected and where the data is being stored.
The Headline Data Catalogue allowed Parkinson’s UK to learn more about who their audience and pinpoint any particularly useful data more easily and accurately.
2. Establishing relationships between the different data sets
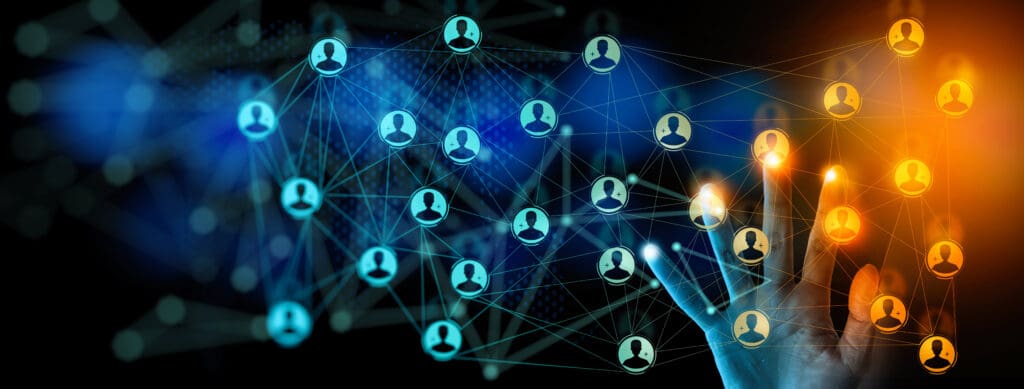
Next, we implemented a Conceptual Data Model, ERD (Entity Relationship Diagram). This tool helps to visualise the relationships between data tables, demonstrating how different sets of data are related. For example, a customer might be represented both in the CRM tool and the accounts tool.
The ERD helped to make sure both sets of data were connected so it was easy for Parkinson’s UK to see the big picture. Being able to see the data like this means it is also easier to spot gaps and errors.
3. Highlighting gaps and areas of concern in the data
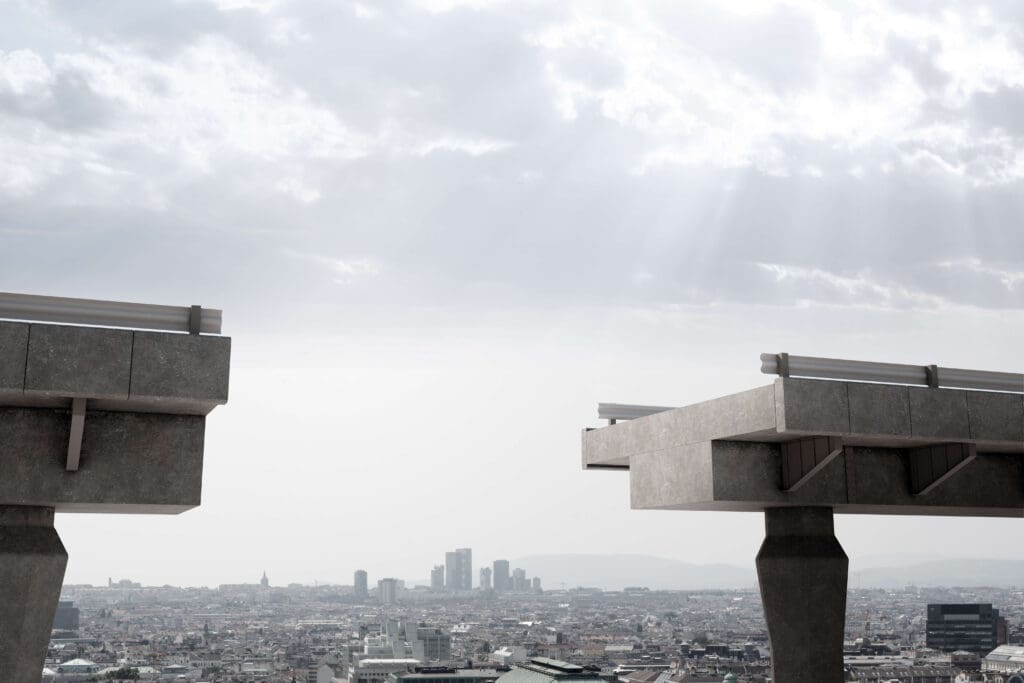
In order to uncover any missing or incorrect data, we carried out an Exploratory Data Analysis (EDA). An EDA is a report driven by deep level data profiling, distribution and trend analysis. The report provided insights into Parkinson’s UK data content, volumes and quality, highlighting any defects, duplicates or inconsistencies.
This report is also useful for drawing attention to anything else unexpected that might influence future decisions, changes or integrations. Fixing duplicates, for example, saves both money on storage space and marketing, as well as improving the communications experience for customers.
4. Creating rules to bring continuity to the data processes
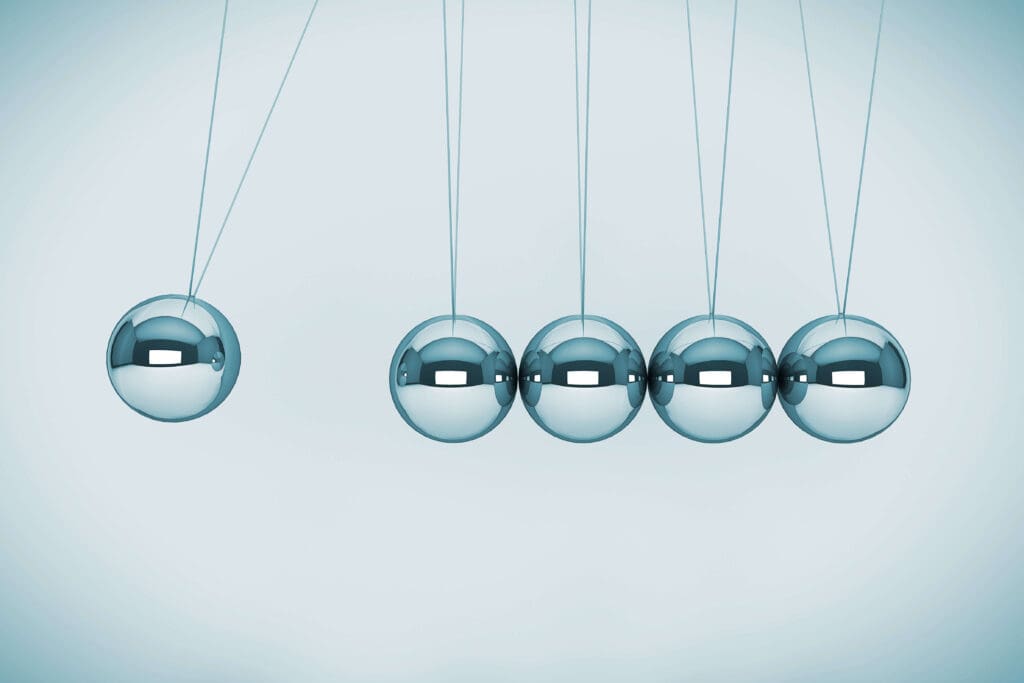
Our next step was to establish how to fix these and to prevent this from happening again in the future. The EDA allowed us to catalogue a list of issues that needed to be resolved through Data Quality Rules (DQR) flags. These issues include, duplications, titles, address, email completeness, and address completeness.
By shining a light on these issues, Parkinson’s UK could take control of their data. They were able to work with their team to establish what rules needed to be in place to bring continuity to their data processes. Not only does this improve data quality and save time in the long run, but it was also useful for building skills within the team.
5. Creating an accessible report, ready to present to the C-suite
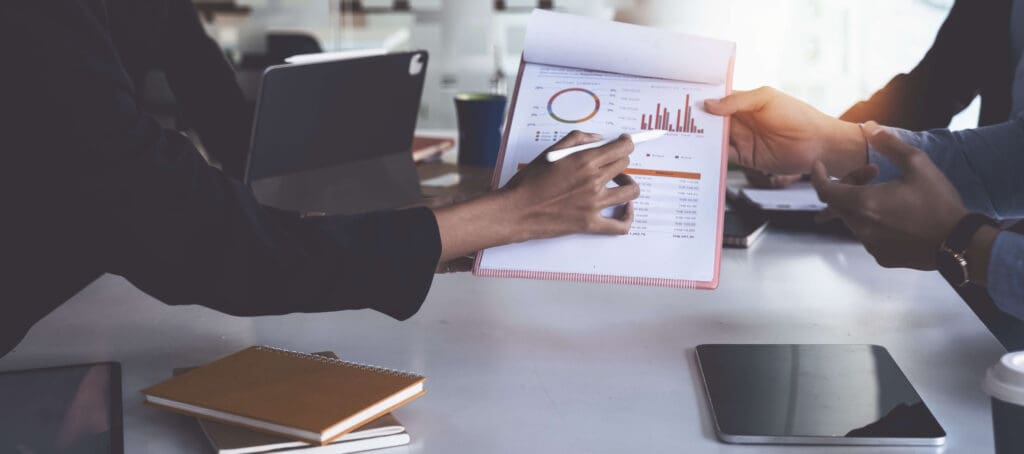
Finally, we finished the landscape analysis with an executive summary report that brought together all the findings. We always make sure our reports are non-technical and readable so that it is clear to anyone in the company. We presented the findings of the analysis back to the team at Parkinson’s UK, highlighting issues around data quality and providing the team with a development roadmap.
The team at Parkinson’s UK were then able to easily take our findings and communicate them to the board members.
A landscape analysis is always the most effective first step to any data project and will pave the way to a successful outcome. In this case, we were able to give Parkinson’s UK a full picture of their data landscape and guidance on how to improve their data quality. We then went on to help Parkinson’s UK prepare for a data migration, implementing a data warehouse as a single source of information.